AI and machine learning in credit control
Corcentric
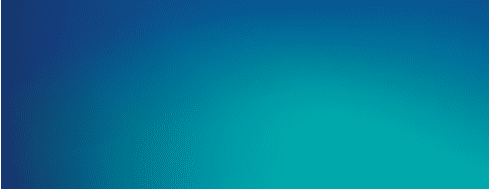
Artificial Intelligence (AI) is poised to transform many digital processes in credit control. In particular, machine learning, as a subset of AI, presents a step-change in credit control processes.
Through the application of machine learning, credit controllers will be able to identify credit risks more rapidly. Moreover, this will guide credit control processes to improve cash flow and limit credit risk.
What is machine learning in credit control?
Machine learning in credit control refers to the use of algorithms and statistical models that computers use to perform tasks without explicit instructions, specifically in the context of assessing credit risk.
Machine learning is an evolution beyond the use of algorithms in business processes. Specifically, it is the process of a machine refining the algorithms it uses in data processing. This process of learning is typically supervised by a human. However, the machine has the capacity to learn and evolve to process data with greater speed and accuracy than its human supervisor(s).
Machine learning is categorized in several different ways, depending on how supervised and reinforced the learning is. Supervision and reinforcement improve the efficiency of the output but require considerable human input. For example, the vast number of humans that manually assist in the learning of driverless cars.
As computing power becomes more readily available, less supervised machine learning becomes more practical, especially with the rise of cloud computing. Deep learning is an advanced subset of machine learning, where the machine learns from various interdependent layers of data rather than the more simplistic models required for traditional machine learning.
How is credit scoring normally done?
Traditional methods:
Traditionally, credit scoring was conducted using calculations based on a set of rules or a model. One of the most notable models used for this purpose is the Altman Z-score, which uses linear discriminant analysis based on several accounting indicators. This approach requires a specific set of accounting entries as input for the calculations. Companies that do not provide this specific set of data are excluded from the analysis, limiting the breadth of the data used in the assessment. Additionally, the rigid nature of these models means that fluctuations in data availability result in exclusions from the analysis.
[Altman et al, 2014]¹.
Current trend with Machine Learning and AI:
In stark contrast, the modern approach to credit scoring leverages Artificial Intelligence and Machine Learning technologies. These advanced systems are capable of dynamically analyzing a wide range of data sets, including both structured and unstructured data. This enables credit controllers to identify credit risks more rapidly and with greater accuracy [Umagba et al. (2023)]2. For instance, machine learning algorithms can consider factors such as payment history, social media activity, and economic conditions, which were previously difficult or impossible to incorporate into credit scoring models.
Moreover, machine learning models are self-improving; they learn and adapt from new data, making them more accurate over time without the need for constant human intervention. This adaptability allows for a more flexible and comprehensive analysis, accommodating fluctuations in data availability without excluding companies from the analysis. As a result, modern credit scoring methods using AI and machine learning achieve a balance, allowing for greater depth of data without sacrificing the breadth of data considered.
Why is machine learning relevant to credit control?
Fundamentally, machine learning enables a far greater degree of flexibility in modeling. Introducing flexibility needs to be paired with an ongoing review of performance and iterative improvements resulting from this. Teaching a machine to modify its models based on results from a feedback cycle like this is where machine learning really comes to the fore.
As machines weigh up the relative impact of various data on credit risk, they can dynamically adjust the weighting from each area. This enables a wide range of different data sets to be used effectively for credit scoring. In conclusion, this means that greater data breadth can now be achieved when using greater data depth.
Initially, such analysis needs to be supervised and reinforced, but over time the machine learns to make its own judgments. Consequently, the complexity of such machine learning approaches can far exceed what is feasible for a human to regulate and supervise.
Invoice payments and credit scoring
At Corcentric, we are particularly excited to see this taking effect through the utilization of invoice payment timeframes and billing interactions. Late payments, delayed responses, and the requirement for payment reminders are all factors that can be utilized in credit scoring – providing greater depth than traditional scoring models.
As sellers shift to electronic invoicing, this data becomes easily available via the e-invoicing platforms. These can be interrogated via API or data export by credit scoring systems to furnish their machine learning processes with this data.
A pincer movement via e-invoicing
On one hand, data from e-invoicing platforms can be used to better inform credit scoring tools. On the other hand, e-invoicing provides early warning signals and better controls over accounts where there is a higher credit risk.
Through a web-based e-invoicing platform, such as Corcentric, users can indicate their intent to pay – even if they are not paying immediately. Combining this with real-time visibility of whether buyers have received, read, or paid invoices gives a better insight into payment status.
Higher-risk accounts can be managed more tightly through e-invoicing. Early payment discounts can be used to incentivize payment. Combined with easy online payment gateways and clear communication processes, e-invoicing equips businesses with the tools to proactively manage their credit risks.
The leveraging of AI and machine learning in the credit control context is not just a trend; they are shaping the future of how credit is assessed. Contact us today to explore how our AI solutions can transform your credit control processes.
- [Altman et al 2014] Altman, E.I., Iwanicz-Drozdowska, M., Laitinen, E.K. and Suvas, A., 2014. Distressed Firm and Bankruptcy Prediction in an International Context: A Review and Empirical Analysis of Altman’s Z-Score Model.
- [Umagba et al. (2023)] Umagba, A.O., Abara, B., Isa, Z., Okoro, E., and Yahaya, M. (2023). “A Multi Class Machine Learning Model for Predicting Credit Default in Credit Risk Management.” MLWA-D-22-00233.