Creating a data analytics approach to fraud detection and prevention
Corcentric
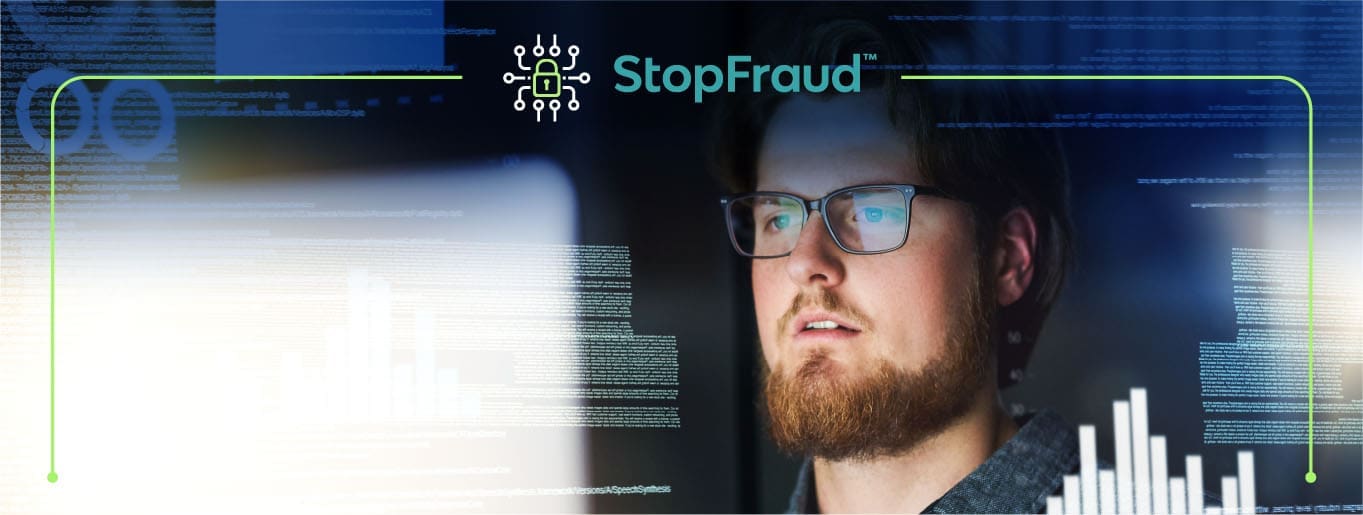
In an era where digital transactions are commonplace, bad actors have become increasingly sophisticated, making prevention a relentless battle. Fraud analytics serves as the frontline in this ongoing war against deceitful activities within systems designed for trust. It marries statistical techniques and cutting-edge technologies like AI and machine learning to uncover irregularities.
Understanding the role of data in combatting fraud is a cornerstone of modern security measures. By tapping into the power of analytics, institutions can not only react swiftly to fraudulent incidents but can also foresee them. As we delve into the benefits of utilizing fraud analytics, see how a proactive and informed approach can transform the landscape of fraud prevention, whether that’s detecting fraudulent supplier information, financial fraud, or using predictive analytics to spot invoice fraud.
Understanding fraud analytics
Fraud analytics represents a sophisticated area of data analysis that integrates traditional approaches to fraud detection with advanced analytics to identify fraud patterns, and predict, prevent, and mitigate fraudulent activities. By applying advanced analytical techniques, organizations can enhance their capabilities to distinguish between legitimate transactions and suppliers, and those that are potentially fraudulent. A key aspect of fraud analytics is its ability to discern suspicious patterns and detect abnormal behavior that deviates from the norm across a variety of data types, often in real-time.
This proactive approach to monitoring and predicting potential fraud allows institutions to respond quickly to potential threats, adapting their strategies to the ever-evolving techniques of bad actors. As a testament to its growing importance, the global fraud detection and prevention market size is expanding rapidly, with projections pointing towards a valuation of $66.6 billion by 2028. This significant surge underscores the critical role analytics plays in today’s battle against fraud risks and amalgamates data science, statistics, and criminal psychology disciplines.
Definition of fraud analytics
Fraud analytics refers to the use of data analysis tools and predictive models to detect fraud patterns, and deter deceptive practices. By scrutinizing data, algorithms can uncover hidden patterns, unexpected correlations, outlier transactions, and networks of suspicious behaviors that might indicate fraudulent transactions. By integrating intelligence from various datasets, fraud analytics creates a comprehensive perspective that identifies anomalies indicative of potential fraud.
For instance, Corcentric’s StopFraud™ validation solution for AP Payments includes a 12-point payment validation program coupled with comprehensive information verification and management.
Statistical techniques used in fraud analytics
Statistical techniques in fraud analytics include a diverse range of algorithms and methods designed to identify irregular behaviors and outliers indicative of fraud. Some commonly used techniques include anomaly detection methods, clustering and classification algorithms, regression analysis, text mining, and predictive modeling. These techniques can help detect strategies used by bad actors by learning from historical data and applying those insights to current activities to identify potential fraud risks.
Role of artificial intelligence and machine learning in fraud prevention
Artificial intelligence (AI) and machine learning (ML) play a critical role in enhancing fraud analytics by enabling more advanced and dynamic analysis of data. Machine learning algorithms use predictive models to identify even the subtlest indicators of fraud through continuous improvement from new and incoming data. AI can automate complex processes and decision-making, reacting to new potential threats in a fraction of the time it would take human analysts. By applying these cutting-edge technologies, organizations can stay one step ahead in detecting and preventing fraudulent activities.
Definition of fraud analytics
Fraud analytics is a process that leverages advanced analytics to identify and combat fraudulent activities. By analyzing large volumes of data, it can detect suspicious behaviors and potential threats, aiming to pinpoint fraud risks before they manifest into significant losses. This analytical technology is particularly adept at uncovering patterns and anomalies that may indicate fraudulent transactions or behavior of bad actors.
A key component of fraud analytics is the use of artificial intelligence (AI) and machine learning algorithms. They enable systems to build predictive models based on historical fraud data to identify potential fraud with greater accuracy over time. These tools are critical in adapting to the continuously evolving tactics of bad actors when it comes to detecting suspicious transactions.
Here is a condensed list of elements associated with fraud analytics:
- Advanced analytics: Utilizing sophisticated tools to process and analyze data
- Artificial Intelligence (AI): Employing AI to enhance learning from data
- Potential threats: Identifying potential risks before they become issues
- Fraud risks: Assessing and managing the risk of fraudulent activities including credit card fraud
- Suspicious behaviors: Monitoring and evaluating unusual actions for potential fraud, such as identifying payment fraud
- Fraudulent transactions: Detecting and investigating transactions that may be fraudulent
Fraud analytics is essential in a myriad of industries, from banking to ecommerce, offering a proactive approach to safeguarding against financial and reputational damage caused by fraudulent activities.
Statistical techniques used in fraud analytics
Fraud analytics leverages advanced analytics to identify and prevent fraudulent activities. Statistical techniques are critical in understanding data patterns and recognizing potential fraud. Techniques such as outlier detection identify transactions that deviate significantly from the norm, indicating suspicious behaviors. Predictive modeling uses historical data to anticipate future fraud risks, employing algorithms to flag potential threats. Network analysis reveals hidden relationships and connections between entities, which is instrumental in uncovering fraud rings and complex schemes by bad actors.
Key statistical techniques in fraud analytics:
- Outlier Detection: Finds abnormal transactions
- Predictive Modeling: Projects fraud risks based on historical data
- Network Analysis: Uncovers patterns of relations and interactions
- Machine Learning: Adapts to new data for improved detection
- Behavioral Analytics: Analyzes user behavior for irregularities
- Time Series Analysis: Evaluates transactions over time for anomalies
These statistical techniques in fraud analytics are essential in minimizing the impact of fraudulent activities and maintaining the integrity of systems by detecting and preventing fraud efficiently.
Principles of data analytics for fraud detection
Fraud analytics harnesses the power of data analysis, strategic approaches, and advanced machine learning techniques to evaluate the likelihood of fraud and bolster fraud detection and prevention systems. The principles behind these analytical methods are grounded in sharp vigilance into the risk of fraud, and the ability to process vast sets of data to uncover anomalies.
One fundamental technique of effective fraud prevention involves anomaly detection, which centers on identifying transactions or data points that starkly contrast with established norms. By recognizing these outliers, organizations can flag potential fraudulent behavior for further investigation. Pattern recognition is another key strategy, where algorithms search for repetitive sequences or relationships within data that may suggest fraudulent conduct.
Fraud detection algorithms rely on a breadth of data analytics tools capable of scanning through and making sense of high volumes of complex data, and are a key component in fraud detection software. This analysis not only pinpoints individual indicators of fraud but also maps out intricate relationships and patterns characteristic of fraudulent networks. As highlighted in The State of ePayables 2024 report by Ardent Partners, when employing these methods, organizations can discern fraud risks with greater precision and efficiency.
Importance of data analysis in fraud prevention
Data analysis plays a pivotal role in fraud prevention strategies by providing a comprehensive view of transactions and user behaviors. As fraudulent schemes become increasingly sophisticated, the ability to parse through and analyze massive datasets is crucial. Effective data analysis aids in constructing robust defense mechanisms that pre-empt fraud by flagging irregularities as they occur.
Through the continuous monitoring and analysis of transactional data, organizations can identify and respond to fraudulent patterns swiftly. This proactive stance is vital, as delayed detection often results in more considerable financial losses and reputational damage. With diligent data analysis, risks are not only mitigated in a shorter time frame but can also be anticipated, with suspicious activities prevented before they result in harm.
The analysis of data for fraud detection includes a variety of techniques such as statistical analysis, predictive modeling, and machine learning—an aspect of artificial intelligence. These methods are applied to vast quantities of transactional data to spot unusual patterns and detect fraudulent transactions that might otherwise go unnoticed.
Techniques in Advanced Analytics | Description |
Statistical Analysis | Examines data to find anomalies outside of statistical norms. |
Predictive Modeling | Uses historical data to predict future fraudulent activities. |
Machine Learning | Automates the detection of fraudulent activity over time. |
Fraud detection systems powered by advanced analytics can monitor and analyze behaviors in real time, flagging any activity that appears suspicious. This proactive approach not only stops fraudsters in their tracks but also aids in building stronger defense mechanisms against potential threats, safeguarding the financial integrity of businesses and their customers.
Key steps in data analysis for fraud detection
Implementing data analysis for fraud detection methods involves several key steps, each integral to developing a robust fraud detection strategy:
- Data Collection: Accumulate comprehensive transactional and behavioral data from various sources to form a solid basis for analysis
- Data Processing: Cleanse and normalize data to ensure accuracy and consistency across different sets, making it suitable for analysis
- Feature Selection: Identify and select specific data features that are relevant to detecting fraudulent behavior
- Model Development: Develop statistical and machine learning models that use selected features to identify potential fraud
- Testing and Training: Test the model against historical data and continuously train it with new data to improve its predictive power
- Real-time Analysis: Implement the model to analyze data in real time, allowing for the immediate flagging of suspicious activities
- Review and Investigation: When potential fraud is detected, perform a manual review to confirm fraudulent activity before taking action
- Feedback Loops: Use the outcome of fraud investigations to refine and improve the analytical models, creating a feedback loop for continuous learning
These steps, when implemented correctly, help establish a comprehensive analytics-based approach to fraud detection that can adapt to evolving fraudulent tactics and maintain the integrity of financial systems.
Benefits of using fraud analytics
Fraud analytics has become an essential tool for organizations across various industries to protect themselves from a plethora of financial risks. By leveraging the power of data analysis to pinpoint fraud indicators, organizations can defend themselves against false transactions, money laundering, asset misuse, data leakage, and privacy breaches. The implementation of predictive fraud analytics allows businesses to develop sophisticated models that assess the risk of future fraudulent activity. These risk scores are derived from patterns found in historical data, which help in formulating strategies to counter potential threats.
The advanced capabilities of fraud analytics also include real-time detection and actionable insights, which are integral in the swift identification of potential fraud. Through intricate pattern recognition and sophisticated data analysis techniques, the technology can promptly flag suspicious behaviors that could indicate fraudulent transactions. This immediate response mechanism is vital in curtailing the spread of fraudulent activities before they wreak havoc on financial systems.
Furthermore, fraud analytics is instrumental in reducing the financial burden of fraud on both institutions and their customers. With early detection of anomalies, the costly effects of fraud can be mitigated, ensuring that the economic impact remains minimal. In addition to protecting financial interests, advanced fraud analytics significantly enhances customer satisfaction. By implementing proactive measures that secure customer assets and sensitive information, businesses not only establish a trusted environment for their clientele but also reinforce their reputation as being safe and responsible.
Early detection of fraudulent activities
The early detection of fraudulent activities is one of the most significant advantages of fraud analytics. By spotting potential fraud as it occurs—or even before it is fully executed—organizations can act swiftly to prevent losses. The real-time nature of modern analytics tools means that businesses are not reliant on periodic reviews or audits, which could delay the discovery of fraud by weeks or months. Instead, continual monitoring and analysis of transaction data allow for the immediate isolation of suspicious activities.
Furthermore, the feedback loop provided by ongoing surveillance feeds into the predictive capabilities of fraud analytics systems. As potential fraud is detected, the system’s algorithms adapt and improve, enabling even more rapid identification in the future. This proactive approach to fraud detection not only saves on resources but also instills a culture of vigilance against fraudulent behaviors, discouraging bad actors from attempting to exploit the system.
Improved accuracy in fraud detection
In the realm of fraud detection, advanced analytics has markedly improved accuracy, enabling organizations to effectively pinpoint and respond to potential threats. Utilizing a blend of techniques such as artificial intelligence (AI) and machine learning, these systems can analyze vast volumes of data to identify patterns indicative of fraudulent activities.
AI-driven models are particularly proficient at detecting suspicious behaviors that deviate from the norm, which are often the hallmark of fraudulent transactions. By continuously learning from new data, these systems become increasingly adept at predicting and preventing bad actor schemes before they inflict significant damage.
Fraud risks are not static, so the agility afforded by advanced analytics is crucial. It empowers organizations to adapt to the evolving tactics of fraudsters, maintaining an edge over potential fraud. Furthermore, the capability to analyze unstructured data widens the net for detecting sophisticated fraudulent activities that might otherwise slip through traditional detection methods.
Getting started with fraud detection
If you are looking to identify fraud in your AP payments, check out the Corcentric StopFraud™ Validation solution: an advanced system designed to prevent fraudulent activities by safeguarding customer and supplier payment information. The StopFraud™ system employs a comprehensive twelve-point protection strategy, including measures such as customer-specific access codes, IRS TIN matching, USPS address verification, and dual-factor authentication. This is just one example of a system that provides peace of mind by preventing fraud before it happens.